Adapting threat models in new environments is a perennial data security challenge. AI presents new challenges that require different approaches to mitigate effectively. Recent research identifies common blind spots, especially for organizations with mature data security programs. Most businesses in regulated industries have data security programs that work well in traditional use cases. However, AI requires an expanded threat model encompassing new areas where controls must be applied and where data may be at risk.
A common challenge with any novel technology is fully understanding its security implications. Too often, enterprises attempt to extend their existing security controls into new environments. As workloads shifted to cloud, legacy security tooling tried to adapt, but cloud scale, automation and abstractions created challenges. Developers could access infrastructure without the guardrails that existed on-premises, creating new security risks. AI presents similar challenges, and it’s doubly important to learn from previous transitions. Not only has AI adoption advanced rapidly, but it encompasses a broad set of users across organizations.
The Verizon AI at Scale study, conducted by 451 Research and commissioned by Verizon, reveals instructive contrasts. In interviews, senior executives express concerns about AI security issues, but many expect that on-premises deployments and existing data protections will manage risk. This contrasts with AI implementors, who expect security capabilities to be the area of greatest change required across their infrastructure in the next two years, topping needs for compute, storage and networking. Security is the only category where all senior executives and AI implementors expect change, and it garnered the highest level of expected change.
To benefit from AI’s capabilities while managing risk, organizations must broaden their security planning. Unlike securing a database, controlling access and authorization for AI requires an understanding of what is being done with data, how it is integrated and the many new pathways it may traverse. Data input controls must integrate more complex context while still protecting against existing attack tactics. Models must be protected in ways that go beyond typical application security. Output data must be correlated with inputs, not only to understand accuracy but to identify abuse.
The architecture of AI deployments presents additional risks that may not be anticipated. When models are strengthened with supplemental data, as in retrieval-augmented generation (RAG) architectures, they can access databases in ways that create risk. Overprovisioning of access is a concern, as a model may need broad data access to accomplish its goals. Prompts to the model could then expose data relationships, such as linking customer identities to order data, that might otherwise have been separated by role restrictions assigned to individual users. Similarly, volumetric or behavioral controls may be bypassed to support the volume of model-generated queries — a measure that no individual user would be allowed to take. For example, no individual user should ever extract a complete listing of customer data, but an AI model might be allowed to.
A major strength of AI applications is unifying large volumes of data from disparate sources. However, an often-unanticipated challenge is the potential for the model to infer relationships in anonymized data. While an organization can make efforts to anonymize data, a model could recreate associations that anonymization was intended to prevent. Masking or field restrictions may be inadequate, and fully synthetic data may be required.
Much like other data sources, AI models intensify risk by concentrating valuable data. Databases, for example, are valuable attack targets because compromise yields a rich trove. Unlike databases, however, AI models are distributed to points of inference to do meaningful work. While perimeter defenses may protect databases, it can be daunting to distribute and manage protections at AI scale.
AI has great potential to improve enterprise efficiency and effectiveness, and its use has become a competitive imperative. Organizations must apply extended security thinking to ensure that implementations don’t become a liability. Effective protections require approaches that fully comprehend the new threat model that AI creates and mitigate the new risks that arise.
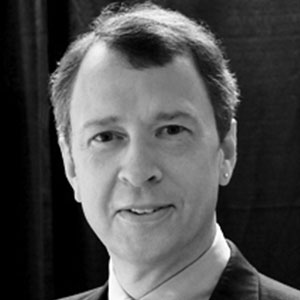
Eric Hanselman, Principal Research Analyst, 451 Research
Eric Hanselman is the chief analyst at S&P Global Market Intelligence. He coordinates industry analysis across the broad portfolio of technology, media and telecommunications research disciplines, with an extensive, hands-on understanding of a range of subject areas, including information security, networks and semiconductors and their intersection in areas such as SDN/NFV, 5G and edge computing. He is a member of the Institute of Electrical and Electronics Engineers, a Certified Information Systems Security Professional and a VMware Certified Professional.
Explore more
Making AI work for your business
Discover strategies for implementing and managing AI securely, to help you enhance efficiency, productivity, growth and innovation.
Part 1 AI infrastructure matters
Discover why scalable, secure, and modular AI infrastructure is vital for successful enterprise adoption and how hybrid models help meet evolving demands.
Part 2 Data security in an AI-driven world
Learn how AI is reshaping data security. Discover new risks, evolving threat models, and strategies for protecting sensitive data in AI environments.