To fully realize the promise of AI, organizations must be able to deliver its benefits to support even the most sophisticated use cases. While many talk about edge capabilities, few have developed the operational capacity to deliver AI close to customers, employees and target users. The performance benefits are significant, and the expanded utility is compelling. Edge AI is seen by many as a key step in the journey to real-time inferencing. The principal challenges at the edge are connectivity and operational scale. The AI application life cycle requires the movement of data, models and telemetry at volumes that are dramatically higher than for traditional applications. The fundamental issue with scaling at the edge is the large number of locations to manage. Intelligent planning and implementation can address these issues, but it’s a challenge that most enterprises are only starting to grasp.
The Verizon AI at Scale study, recently conducted by 451 Research and commissioned by Verizon, revealed insights regarding enterprises' current edge AI implementations and what they hope to achieve in the future. Edge is a new infrastructure element for most, with only one in three respondents leveraging edge computing at all, and just 2% deploying AI applications there. In broad market studies, just arriving at a consistent definition of the edge can be complicated. Importantly, there is strong agreement that much of AI’s future value will manifest at the edge.
One factor influencing low levels of AI edge use today is self-limitation by enterprises. Where there isn’t capacity or connectivity to support AI use cases, enterprises aren’t exploring them. This misses significant opportunities that could drive competitive positioning, and respondents are aware of that potential. The real work of AI is done through inferencing, and the ideal location for inferencing is where AI outputs will be used. When quick response is critical, the edge will yield the fastest returns. Respondents identified many use cases that would benefit from real-time inferencing at the edge. In healthcare, clinical AI applications and emergency care triage topped the list. In manufacturing, sophisticated quality and process control were key. Utilities respondents cited distributed grid control and infrastructure operations. All these depend on placing AI capabilities where data is generated.
Effective AI at the edge requires both compute capacity and connectivity at levels that exceed traditional edge applications. Uses such as machine learning-based machine vision in manufacturing are widespread and can function well with limited connectivity. Retraining is infrequent, and there is often little coordination of operations or data sharing between sites. However, for an AI deployment in a similar setting to incorporate adaptive analytics and supply chain optimization, it would require enhanced connectivity to coordinate between locations and manage the operational aspects of the deployment. Operational telemetry is fed back to monitor and retrain models, and updated models are distributed regularly. Study respondents expressed concerns about latency in AI results limiting certain applications, whereas using local AI deployments can overcome latency caused by physical distance.
Edge AI implementations can have additional infrastructure benefits. Edge AI can distribute processing loads by performing analytics locally, reducing the burden on centralized resources. AI engines at the edge can help to manage the volume and cost of edge data returned to datacenters or cloud by turning raw data into events and metadata.
Operational scaling for edge AI requires automation to deploy and manage model life cycles. The effort of deploying and securing models in multiple locations could overtax IT teams. Many enterprises are striving for increased automation but have yet to master it. Notably, survey respondents identify automation as both an expected benefit of AI and a necessary component to support AI operations, illustrating the need for a comprehensive, multifaceted approach to edge AI deployments.
The benefits of edge AI are worth the investment in careful planning. Building a foundation of connectivity and operational scalability can help to ensure successful results and a fulfillment of AI’s promise.
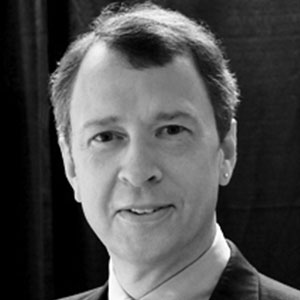
Eric Hanselman, Principal Research Analyst, 451 Research
Eric Hanselman is the chief analyst at S&P Global Market Intelligence. He coordinates industry analysis across the broad portfolio of technology, media and telecommunications research disciplines, with an extensive, hands-on understanding of a range of subject areas, including information security, networks and semiconductors and their intersection in areas such as SDN/NFV, 5G and edge computing. He is a member of the Institute of Electrical and Electronics Engineers, a Certified Information Systems Security Professional and a VMware Certified Professional.
Explore more
Making AI work for your business
Discover strategies for implementing and managing AI securely, to help you enhance efficiency, productivity, growth and innovation.
Part 1 AI infrastructure matters
Discover why scalable, secure, and modular AI infrastructure is vital for successful enterprise adoption and how hybrid models help meet evolving demands.
Part 2 Data security in an AI-driven world
Learn how AI is reshaping data security. Discover new risks, evolving threat models, and strategies for protecting sensitive data in AI environments.