As organizations step up to higher levels of maturity in AI deployments, the requirements for AI-capable infrastructure become more critical. To move from early experiments to broad adoption, full AI application life-cycle capabilities require not only better data security controls, but also the capacity to deliver data when and where it’s needed. In AI applications, data is the raw material from which functionality is built. To fully realize the value of AI, organizations must build a data pipeline in much the same way they’ve built application development pipelines, and they need infrastructure and connectivity to support it.
A recent study conducted by S&P Global Market Intelligence 451 Research and commissioned by Verizon reflects shifts in organizational understanding, expectations and plans around AI infrastructure. As enterprises deepen their understanding of the infrastructure required to support AI, their focus evolves. Those in the early, experimental stages of AI implementation often underestimate the importance of modularity and scalability. They may optimize their infrastructure to support a single model for a specific use case.
While this may be sufficient for small-scale experiments, organizations report various challenges as they pivot to enterprise-scale use cases. Major issues center on infrastructure flexibility and scalability, security integration and hybrid readiness. Flexibility concerns are driven by a realization that the model that drives a pilot project may not be the same model used in production. With the rapid evolution of model capabilities and supporting AI architectures, organizations will use a variety of models over the life of a project. It’s also important to address the full model life cycle, in which deployment and monitoring are as critical as training. AI infrastructure must be flexible enough to accommodate these new patterns.
The data security requirements for AI are much broader than those in place at many enterprises. Study participants in regulated industries were more likely to have mature data and infrastructure security protocols, but even these were seen as insufficient to secure complex AI environments which require additional data processing and cleaning as well as new protections around APIs, prompts and outputs.
Many organizations start their AI journey with cloud-based experiments, while some choose on-premises infrastructure for sensitive projects. Wherever they start, most AI deployments grow to include cloud and hybrid infrastructures to balance cost, security and performance. New models, services and capabilities are constantly released, and this can provide important opportunities to improve AI functionality and effectiveness. For hybrid infrastructure to work effectively, organizations found that they needed not only cloud resources, but also performant network connections to link their data resources to AI workloads. Hybrid environments can be complex to manage, particularly if they are not designed and implemented thoughtfully. However, integrating security and network planning can simplify hybrid operations and overcome many of the challenges that respondents identified.
One complexity that the study revealed is potential mismatch between executive-level understanding of the state of AI infrastructure and the reality facing AI architects and implementors. The needs of production AI environments are very different from those that support early pilot projects. While a pilot project can be effective at refining use cases, the shift to production demands a change in mindset and infrastructure approach. A gap in understanding of infrastructure needs can put important AI projects at risk. Budgeting issues are the leading cause of AI project failure, according to a recent 451 Research Voice of the Enterprise AI Infrastructure study. Clear organization-wide communication is necessary to assure successful AI projects in the long term.
AI can deliver powerful benefits across many use cases, but it requires careful planning and new ways of thinking about infrastructure. Starting with a foundation that is modular, interconnected and scalable will help organizations manage costs and improve the chances for AI project success.
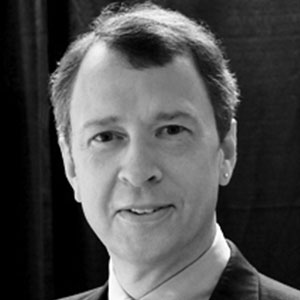
Eric Hanselman, Principal Research Analyst, 451 Research
Eric Hanselman is the chief analyst at S&P Global Market Intelligence. He coordinates industry analysis across the broad portfolio of technology, media and telecommunications research disciplines, with an extensive, hands-on understanding of a range of subject areas, including information security, networks and semiconductors and their intersection in areas such as SDN/NFV, 5G and edge computing. He is a member of the Institute of Electrical and Electronics Engineers, a Certified Information Systems Security Professional and a VMware Certified Professional.
Explore more
Making AI work for your business
Discover strategies for implementing and managing AI securely, to help you enhance efficiency, productivity, growth and innovation.
Part 1 AI infrastructure matters
Discover why scalable, secure, and modular AI infrastructure is vital for successful enterprise adoption and how hybrid models help meet evolving demands.
Part 2 Data security in an AI-driven world
Learn how AI is reshaping data security. Discover new risks, evolving threat models, and strategies for protecting sensitive data in AI environments.